Interview conducted March 6, 2013
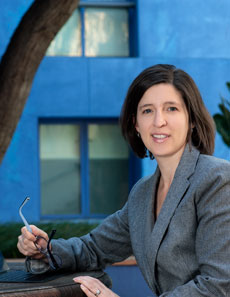
If federal tax rates change, how will that affect a firm’s production decisions? Engineers develop a new drilling technology: What impact might that have on global oil prices? Suppose American teenagers suddenly decide that balloons are very, very cool. Will that significantly alter U.S. helium reserves and extraction?
Such problems—how will an equation’s variables respond to a shift in their economic environment?—are solved with “comparative statics,” a type of analysis that compares outcomes before and after an external change to an otherwise untouched (that is, static) model.
The questions may sound simple, but solving them with precision long required an unwieldy freight of questionable assumptions and abstractions. Stanford economist Paul Milgrom and colleagues improved the process considerably in the early 1990s with “monotone” comparative statics, showing that fewer assumptions were necessary.
Then, in 1994, Milgrom’s graduate student Susan Athey advanced into a far more complex realm: uncertainty. Forecasting in an uncertain world is intuitively difficult, and mathematically the puzzle seemed “just too hard” to Milgrom and others. But Athey solved it, identifying the surprisingly small number of assumptions about risk preferences and types of risk that would “guarantee robust...predictions” in the context of uncertainty.
Athey now refers to this methodology as a simple “black box,” but her elegant solution astounded her colleagues. “I remember being just totally stunned,” Milgrom said years later, in a Wall Street Journal interview.
In 2007, this and other research earned Athey the John Bates Clark Medal. She was the first woman to win the prestigious honor, then given every other year to the best American economist under 40.
The Clark citation noted that Athey’s “powerful techniques...have been profitably used in applied problems.” Portfolio investment decisions, for example, pricing and production choices by risk-averse firms, a worker’s quandary about going back to school, everyone’s dilemma about spending or saving—difficult choices seemingly swamped by uncertainty—are all now approachable due to her research.
Now back at Stanford after professorships at MIT and Harvard, Athey continues to develop powerful tools, make empirical discoveries and foster theoretical advances. She’s a pioneer in the economic analysis of auctions. She uses game theory to understand decision-making by businesses and central banks. She has analyzed mentoring and diversity, how staff training and new technology jointly raise productivity, and the Internet’s impact on print media. In the following conversation, Athey touches on a number of these topics and others, always bringing greater certainty to complex realms in economics.
Forestry Auctions
Region: Let me begin with your work on forestry auctions. You wrote a paper, published in 2011 with Jonathan Levin and Enrique Seira, which compared revenue outcomes for open- and sealed-bid auctions. It reexamined Vickery’s 1961 work. Would you describe that work for us?
Athey: Sure. Our work, as you mention, departs from this famous and extremely surprising theorem of Bill Vickery that was Nobel prize-winning research. His basic theorem says that these two different ways of auctioning anything, like timber, under some conditions will yield exactly the same outcomes.
One way is an open outcry auction, where bidders get in a room and keep outbidding each other until the last bidder drops out and the auction ends. The second way is a first-price, sealed-bid auction where the bidders submit their bids in writing. The highest bidder wins and pays his bid.
These two auctions are quite different. In the first, the winner pays the price at which the last competitor drops out, so that the second-highest bidding competitor determines the price. In the other one, the winner pays exactly how much they bid. What’s really surprising is that in theory those two methods produce exactly the same outcomes.
Region: But you looked at data, as well as theory.
Athey: Yes. The paper picks up from the fact that in practice the assumptions of that theory don’t hold exactly. What kind of auction format you use can matter if you have asymmetric bidders. If you have, for example, small bidders who on average have lower values for the thing that’s being auctioned, they can be discouraged from even entering an open auction. They know if they show up, another (larger) bidder can just outbid them.
In contrast, in a first-price, sealed-bid auction, they can hope that the strong bidders might shade their bids a lot, trading off a reduced chance of winning the auction against paying less when they do win. So the small bidders have a chance to sort of “sneak in” and win the sale, even if they don’t have the highest value for the object. As a result of those kinds of dynamics, you expect that more small bidders will show up at a sealed-bid auction.
Region: So it changes participation.
Athey: Yes. And so our paper was trying to document both the effects of the asymmetries on what happens in the auction, conditional on who shows up, but especially whether the participation effects were actually important. One of the interesting findings was that participation is a more important factor than what happens once you get to the auction. So if you’re thinking about how to design an auction, or how to design a market more generally, even though it can be tempting to focus on what happens once the people are in the room, it can be more important to start with designing your marketplace to get people to come, to start with.
This insight is one that I’ve brought to other settings. I think, for example, it applies in online auctions. When a large company like eBay or an online advertising firm is designing its marketplace, for example, it can be more important to design your marketplace to attract bidders and make sure they’re there to participate than it is to try to extract every last cent out of them once they get there. If potential bidders are not making enough profit to make it worth their time to come, they won’t come. And thin markets can be much more problematic.
Position Auctions
Region: This leads to your research on position auctions. You’ve done a lot of that work as a consultant with Microsoft, I believe.
Athey: That’s right.
Region: And your research has modeled position auctions, initially as one-sided markets, and later, in your Quarterly Journal of Economics paper with Ellison, as two-sided markets, where the search engine is the market maker between the consumers and the advertisers, just as a credit card company brings together retailers and buyers.
Athey: Right.
Region: It’s intriguing and complex as a two-sided market. But also intriguing in that these auctions are a very new market—so it’s liquid, it’s dynamic and there’s a ton of new data coming in every day. It’s also a growing market, generating a lot of revenue.
What have you discovered about position auctions in terms of basic findings and how best to design them?
Athey: I started working on Internet search advertising auctions from a theoretical perspective, and then I got the opportunity to work with Microsoft to design their real world search advertising auctions. I should say that economists who consider themselves market designers are really attracted to opportunities to take theory to practice and to do the engineering of making a market work. So this was a great opportunity.
Let me start with the theoretical side. For the QJE paper we wrote together, Glenn Ellison and I observed that the first couple of theory papers that tried to analyze Internet-advertising auctions really focused on the advertising side of the market.
Region: For clarity, these are auctions for ad location on a web page, often seen in the right-hand margin, correct?
Athey: Exactly. So when you do a search on an Internet search engine...
Region: Whether it is Bing or Yahoo or Google...
Athey: Yes, Bing, Yahoo or Google. Usually you’ll get some ads at the top of the page and some ads on the side. The top positions get a lot more clicks than the bottom positions. And because clicks from users are valuable, advertisers are generally willing to pay more per click to get more clicks. So you might pay a lot per click in order to get the top position and get a lot of clicks, or an advertiser can choose to pay a lower price per click, but then they don’t get as many clicks.
So the first couple of papers to try to tackle this problem started at a very natural place, which is to say, imagine we have these positions on the page. The higher ones get more clicks. The lower ones get fewer clicks. How will bidders bid in this auction? And the auction rules—well, actually it was a new set of auction rules invented by the companies in this industry—were designed so that bidders’ prices were determined by the bids of the bidder below.
What Glenn and I noted was this focus on just the advertisers’ side of the market left out some really important aspects of the market design. In particular, we wanted to incorporate the fact that users are going to be more likely to click on ads, and more willing to keep clicking on ads, if they believe those ads are high quality.
We were partly motivated by the fact that at the time, in the mid-2000s, a lot of Internet search advertising was really fairly poor and irrelevant. You might have a lot of ringtone ads, say, or ads for firms that weren’t really selling what you were looking for, but were trying to be misleading.
Region: So they were a waste of money for the advertiser and of time for the consumer.
Athey: Those ads weren’t really generating a lot of value for the advertiser, and the harm to the user side of the market outweighed the benefits to the irrelevant advertisers. Yet it can be very challenging for a search engine to decide to remove those ads because, in the very short run, those ads are making money. Your first-pass intuition might say that if you take an ad away, you can’t possibly make more money.
So what we needed was a model that incorporated the fact that you can, in fact, make more money by showing fewer ads. And that’s because we expect consumers to update their beliefs about the quality of the ads and be willing to engage more. So we presented a full equilibrium analysis of both sides of the market. It incorporated the advertiser-bidding behavior as well as the consumer-clicking behavior and then looked at questions about market design from that perspective.
Region: And that enabled you to evaluate the distribution of benefits among those three parties: search engine, advertiser and consumer.
Athey: Exactly.
Region: Meaning that auction design therefore affects overall welfare—not just advertisers’ welfare?
Athey: Absolutely. Generally, there’s going to be a trade-off between efficiency and revenue. The auction designs that make the most money for the auctioneer are generally not going to be the ones that are maximizing welfare.
But we showed the somewhat surprising result that in this particular model, there was not a trade-off between overall producer welfare—that is, advertisers plus the search engines—and consumer welfare, but rather that those were proportional. That was one of the insights into figuring out, well, how many ads should you actually show?
And the reason those things moved in sync, if you like, is that when you show fewer ads and improve the quality of ads, the equilibrium rate at which consumers increase their clicking is proportional to the improvements in quality you’re providing, which are, in turn, proportional to the surplus that’s being created for advertisers.
This doesn’t mean that’s what a profit-maximizing search engine will do, however, because a profit-maximizing search engine cares how much surplus the advertisers get versus the search engine. As a result of that, a monopolist search engine will tend to raise reserve prices [meaning the lowest price they’ll accept] too high in order to extract more surplus from the advertisers even if it means eliminating ads that the consumers might have liked to see.
In contrast, a competitive search engine—one that’s competing for advertisers and users—will be more likely to choose the welfare-maximizing point.
A more realistic model would also incorporate the other content that gets crowded off the page by the ads; such a model would be more likely to see a monopolist search engine put up too many ads relative to what consumers would like, but again competition would typically push a firm closer to welfare maximization in order to keep both sides of the market participating.
Regulating Internet Search
Region: There have been discussions in Europe, specifically concerning Google, about the possibility of regulating Internet search. Do you have any thoughts on that question, given what you’ve just said about monopolistic practices by search engines?
Athey: One argument that could be made about Internet search engines, a naïve argument, would be that because they’re auctioning off positions, and prices are determined by the outcome of an auction, that competition doesn’t affect prices or advertiser welfare.
Now, advertisers typically disagree with that argument. This theory we just discussed would help interpret why advertisers believe that competition does matter. First of all, when you realize that a search-advertising platform has choices to make, like reserve prices, that affect the distribution of welfare, then it’s natural to realize competition will induce them to put less weight on their own profits and more weight on the welfare of the participants they’re trying to attract to the marketplace.
So it’s really a theory for understanding why this market is no different than other markets where competition causes firms to put more weight on their customers’ welfare and less on their own profits. In a multisided market, though, sometimes the complexities obscure the fact that basic economics still applies. [Laughter.]
The Auction Continuum
Region: You’ve done a great deal of work on auctions generally, in settings as different as forests and the Internet, and ranging from very basic theory to applied research, and very empirical studies. As you’ve written, “It’s possible to study auctions from pretty much every point on the basic-to-applied continuum.” Why does that continuum appeal to you, and do you tend to prefer one over another—theoretical research more than applied, for example, or vice versa?
Athey: I think that the one common theme that unites many people interested in designing markets is that they want to design a market that works. So you’re motivated by the outcome: building something. And so, just like anything you would build, you need a theoretical framework to come up with a good design. And you also need to have evidence about whether that design will work.
So in some sense, solving the problem requires all of the tools. Personally, I enjoy, for different reasons, all of the different ways of approaching a problem. It’s therefore very appealing to do research that brings all those skills to bear.
I think when you approach a new design problem, the space of things to consider is so large, and the set of complexities is so large, that without a clear theoretical framework, you’ll just get lost in the weeds and you won’t really know what’s important. The theoretical framework helps you understand what to abstract away from and what to focus on.
But once you’ve gotten there, the answer to most theoretical questions is, “It depends.” [Laughter.] One choice might be better if certain things about the world are of a certain magnitude, and if the magnitudes are different you might make a different choice. And so the empirical measurement...
Region: Helps shape the theory as well.
Athey: Well, first, the empirical measurement allows you to quantify the size of competing effects and determine what the best choice is. But, yes, along the way the empirical work may also show you that some effect that you’ve abstracted away from or you thought was small is actually big, and that can motivate changes in the theory. Or you might see people behaving in ways that are completely inconsistent with the theory. And then you need to modify the theory, either the theory of the game or the theory of the motivations of the agents or perhaps the theory of the computational constraints that the players are operating under.
For example, in Internet search advertising, you have a nontrivial fraction of your revenue coming from advertisers or campaigns who receive less than 100 clicks per month. If clicks are on the order of a dollar or so, they’re not doing a large amount of business with you. And, of course, they’re also not receiving a lot of data about the performance of their campaigns.
In that environment, it’s not realistic to expect that they will spend the time and effort to fully and rationally respond to market design changes that you make. They might respond very slowly. They might not respond at all until one day they wake up and decide they don’t like your system, and then they might quit. But it would be hard to know why they quit because they’re responding to something you did six months ago.
But you also have hyperrational bidders. Large Internet retailers will have teams of people and computer algorithms finding every penny of profit opportunity and responding instantaneously to small changes.
So you have this huge range of behavior, all or most of it rational in some sense, but very different in terms of its responsiveness to changes in economic primitives. To understand how to manage that marketplace you really need to account for the diversity and heterogeneity, and the only way to learn about that is to do empirical studies, so you don’t simply assume their behavior, but really learn what their behavior is.
These observations motivate a research project I’m working on right now, to try to estimate from data what kinds of objectives advertisers are considering, rather than assuming that they’re all hyperrational and hyperattentive moment by moment.
Region: Perfect economic agents.
Athey: Right, well, they’re all economic agents. They’re just agents with different costs of time and economic incentive to optimize their bidding.
Collusion and Antitrust Policy
Region: In several papers, you’ve studied the relationship between firms that have a profit-maximizing incentive to collude and antitrust policy that seeks to curb collusion in order to protect consumers from price-setting and restricted output. In a paper with Kyle Bagwell, you wrote that under certain conditions, “antitrust policy can have perverse consequences.” You found, I believe, that in particular circumstances, policies restricting communication among firms can actually reduce consumer well-being.
Could you explain that result? What are the potential benefits of collusion among firms? And do you know if this finding had any effect on current antitrust policy, in the United States or elsewhere?
Athey: Sure. Well, to start with, I wouldn’t recommend that all prohibitions on communication be lifted. This research was highlighting that once firms are colluding, that they would collude more efficiently through information exchange.
Consumers would certainly be better off if you can break down collusion, and collusion may very well be more likely to break down if it’s less efficient because it’s providing less profits to the firms. So I don’t actually recommend eliminating those policies.
But really the focus of that work was on the role that private information plays in cartels. The idea is that a naïve cartel might just divide up market shares or fix prices. And that’s bad for consumers because it leads to high prices. But it’s also bad for productive efficiency, because it’s not responsive to market conditions.
What is the beauty of competition? Low prices for consumers, but also allocative efficiency. Getting the production by the right firms at the right times. If one firm gets a good deal on input costs, they should cut prices, which will also shift all the production to them, which will lead to lower average production costs.
One of the less-emphasized costs of cartels is that they don’t have that kind of responsiveness, which also feeds into incentives to reduce costs and so on. Collusion has lots of consequences on the production side.
Our research was about how firms might try to get around that through more sophisticated collusive agreements. We were partly motivated by some of the sophisticated international cartels that did, in fact, have mechanisms in place to try to be responsive to some kinds of market conditions.
One of the things we show along the way is how communication can help those cartels achieve efficiency and that if they try to get there without communication, they will generally be less efficient and also potentially have more risk of the cartel breaking down. So the cartels may then end up with a less efficient outcome as a result of the restrictions on communication.
But the ideal world, for welfare, is that the cartel breaks down altogether. So really, from a policy perspective, I think that my work is better used to help understand what we might see firms do, why we might see them take antitrust risk by communicating, and to interpret the findings of when you break up a cartel or when you look at evidence about a cartel. It’s an example of looking at the richness of real-world behavior and trying to modify theory to incorporate and explain that richness.
Discretion in Monetary Policy
Region: Let me push you in a different policy direction now: monetary policy. I’m sure you’re aware that the Fed is trying to refine its communication policy toward greater transparency and effective forward guidance. In 2005, in Econometrica, you published a paper on the “optimal degree of discretion in monetary policy.” In it you used some of the same tools you did in your paper on collusive firms and antitrust policy: mechanism design, game theory and the role of private information. [See “Veil of Discretion” in the June 2004 Region.]
Would you briefly describe that paper and its results? I believe you recommended something akin to inflation targeting as optimal policy. And also, could you share your thoughts, given this work, on the evolution of Fed policy since the financial crisis?
Athey: Let me start with the paper. Like many economic theory papers, it tries to isolate just one aspect of the problem. It’s not attempting to provide a holistic solution that incorporates all the costs and benefits, but rather to really bring one particular trade-off into really sharp focus.
The trade-off we were looking at starts by considering the motivation for allowing the Fed to have discretion. Why not just have a mechanical policy?
Region: A monetary policy rule.
Athey: Right, why not just have a rule? A big motivation for having a Fed is that we need smart people who either have access to special information or might have a special ability to aggregate lots of different information and understand it in a deep way, and that could result in beliefs about what’s best for the economy. And further, that combination of information and expertise could give us a policy more tailored to particular circumstances [than a simple monetary rule would].
If you believe that that motivation is there—you know, perhaps after the financial crisis, things have changed; the world you had before isn’t the same as the world you have after. So you need the discretion of a privately informed expert individual.
If you hold that belief, then you have to immediately confront the fact that that entity would have an incentive problem, which is that they would like to take advantage of people’s expectations in order to help the economy grow. So they would like to do a surprise inflation in order to stimulate the economy. Of course, as long as they have the ability to do that, it’s going to be very tempting to say that circumstances dictate that this is a good decision.
So, then you’re confronted with the problem of how to provide good incentives to this institution to which you’ve granted discretion. How can you rein them in? And the result of our paper is that it’s actually extremely hard to provide good incentives to an institution that’s out there trying to maximize welfare.
If you imagine that you have a benevolent Fed, so they’re trying to do the right thing, but they realize that they can, in the short-term, abuse their discretion, which will then lead to higher inflation expectations in the long run. It’s very difficult to provide those incentives without causing more harm than help.
There’s a cost-benefit trade-off to discretion, where you might think the resolution would be, “it depends.” The benefit of discretion is that you can fine-tune policy using the Fed’s private information. The cost is that you have to have some future consequence from inflation today; otherwise you’ll be tempted to do too much.
In our theoretical model, we show that the costly incentives that you have to provide to keep the Fed from overreaching are proportional to the benefits, and because of that proportionality, you can say that actually the cost almost always outweighs the benefits.
So the theory weighs against full discretion and maybe suggests that putting some constraints on is a better approach.
But I should emphasize that the model just focuses on one aspect of the problem, and I actually don’t have an opinion about what the Fed should do now.
Region: Fair enough. You’re one of the few without. [Laughter.]
Organizational Complementarities
Region: Robert Solow famously said that “computers are found everywhere but in the productivity data.” [See September 2002 Region interview.] But when you examined the “Enhanced 911” program, you found productivity gains from that new technology that were literally lifesaving. To do that you developed a new methodology to analyze “organizational complementarities,” or interactions among practices adopted by organizations. Can you describe that methodology and tell us a bit about your findings?
Athey: Sure, so I did this work in the 1990s. Going back to that time, one puzzle was that it was very difficult to actually see evidence in the data that computers had really done very much for the economy.
Region: Right, and Solow wrote that in ’87.
Athey: Yes. Computers, on the one hand, seemed to be an amazing innovation, but on the other hand, by the mid-1990s, what had they really accomplished? One reason it was hard to measure the effect of computers was that organizations were often choosing to change in other ways when they adopted computer technology. In manufacturing, for instance, they might move to a different kind of automation and, at the same time, use different methods to motivate the employees and to organize their work.
Region: Conditions weren’t the theorist’s “everything else equal”; things were changing more or less at the same time.
Athey: Exactly. And not just that things happened to be changing but that, in fact, certain types of practices tended to go together with technology. So if the cost of technology fell, you would choose to simultaneously change multiple other aspects of a firm’s organization and HR practices. So, in fact, you shouldn’t expect to see out there in the world a lot of people adopting technology without making other changes. That means you would be unlikely to have a lot of data about such firms.
If you want to isolate and measure the effect of just the technology, you’d like to see the technology change and nothing else change. But once you think about the organization’s choices, you realize the ones who adopt a technology change and don’t change anything else are actually probably a little bit weird and may not be representative for measurement.
This introduces a very large challenge for measuring the effects of technologies that also affect organizations. The methodology that I developed tried to take very seriously the idea of these coordinated changes, by figuring out that if you did have data about firms’ decisions about technology as well as their organizational practices, and you tried to do statistical analysis on it, what kinds of biases would come out? And under what conditions would your naïve results give you overestimates or underestimates of the benefits of technology?
The first part was just saying, basically, this is really hard: If you take the naïve approaches to the problem, you’re not going to get the right answer. But you might be able to use what you get to provide some directional insight if you can get some particular kinds of supplementary information about what’s actually happening in that particular industry.
So I identified the information you would need to have to tell you whether you’ve got a lower bound or an upper bound of the benefits of technology. I then introduced a methodology that could, under some conditions, solve these problems, but the conditions were quite difficult to meet: There might not be many settings that really had the right data structure to measure the effects.
One type of ideal environment would be a situation where the costs of the technology are changing at the same time that perhaps some labor regulation was happening. So, for instance, you might have some states introducing a labor regulation that makes certain policies difficult or some states having different types of union regulations. Using that kind of data, you can start to disentangle the effects.
Region: So you’re looking for the right sort of natural experiment?
Athey: Right. I identified the kinds of natural experiments that would allow you to disentangle the effects while being cautious that those experiments would be difficult to find because you basically need two coinciding natural experiments in different dimensions if you want to measure the effects of two complementary inputs.
Region: And you looked at this in a program called “Enhanced 911.” Where was that being implemented?
Athey: I looked at the effects of Enhanced 911 in Pennsylvania, and there I used the fact that a new technology was introduced, so the prices were falling and the availability changed during my sample. Because of that, I was able to see the same organizations before and after the adoption of this technology.
I also had a simultaneous introduction of a certain set of training protocols for te 911 operators. Because both of those were newly introduced, I did have two different natural experiments coinciding.
The training was called EMD; it was a protocol for 911 operators to give instructions on CPR and other types of first aid over the phone. Topical in the last week.1
Region: Yes, really.
Athey: Without those instructions, the operators really weren’t able to help a caller very much and there were concerns about liability and so on. In this Enhanced 911 paper I was able to show and measure the productivity impact of new technology and measure its impact on people’s health outcomes.
But I think that, still to this day, it remains difficult to accurately measure all the benefits that computers bring us. Some of the benefits are priced, but how do I measure [the benefit of] the fact that it used to take a minute for a web page to load on your mobile device and now it takes 10 seconds, and so you’re able to get the information a little bit faster? As a result maybe you get to the restaurant faster or you choose a better restaurant for you. A lot of consumer surplus is generated in terms of getting a better match or a more timely match between what you, the consumer, want and what’s out there.
It’s the same thing with a search engine. Without a search engine you would go to the same old website you already always go to. With the search engine you might go to a new website you’d never heard of, and presumably you’re happier as a result of having more choice, but how much happier? How do I measure how much surplus is created by the better matching?
The E911 case was a specific example where getting the service customized to you—the ambulance driver knows where to go the second you call—saves you time, and in the particular case of heart attack patients, we can translate minutes into mortality probabilities. And that gave us a welfare benefit of people’s time. But most of the time faster access to information is not life and death, which is good for the consumer, but not so good for the econometrician trying to measure it!
So comparing today to the 1990s, I think, there are very few people left who are skeptical that computers have led to fundamental changes and have impacted our economy. But we still don’t have that many measures of it. [Laughter.] Now it’s just so obvious that we don’t question it, but actually measuring it remains hard.
Building Better Models
Region: You’ve just given a great example of the difficulty of building a good model.
Some of your earliest work sought to help economists in that regard, by taking advantage of what economists call “monotonicity assumptions.”
Athey: Right, it did.
Region: And the Clark award certainly recognized that. But, “monotonicity,” “single crossing properties,” “hypermodularity”—these are difficult terms...
Athey: Supermodularity.
Region: Supermodularity, thank you—well, that illustrates my point, no? [Laughter.] For me, clearly, and perhaps nontechnical audiences generally, this is pretty impenetrable work. The terms themselves are daunting.
Yet it’s landmark research that has allowed economists to simplify models by clarifying which assumptions are essential and which aren’t. In the words of the Clark award citation, by “exploit[ing] monotonicity assumptions” your work has “...facilitate[d] the development of more robust empirical results” and “powerful techniques.”
Can you explain that outcome? How does that body of work help economists develop better methods and models, and more robust results?
Athey: You can think about a hierarchy of economic theory. Some work is really intended to improve the tools that people use, and other work is about using those tools to solve a problem. The work on monotonicity was about trying to develop tools that allow people to focus more on the problem and less on the tool.
Part of this work was just noticing a pattern: that lots and lots of papers trying to solve problems would start by having to establish certain properties of the problem in order to make it tractable to analyze.
What I tried to do was find those common themes and simplify everyone’s work by allowing them simply to formalize a set of arguments that people were having to make over and over again, each depending on the special features of the problem. And, instead, allow them to just establish some simpler conditions and then apply my results to say, OK, the things I want to do are automatically going to follow.
So you can think of having 20 different specific problems. And you have 20 researchers really interested in each of those problems. Each researcher has to spend a lot of time dealing with little technical details that can be quite frustrating and hard that distract them from the actual problem. I kind of packaged all that up in a nice “black box” where they could simply establish the things that were more intuitive, reference my results and then move on with the economics of the problem.
Region: That’s a very simple and modest way to put it. Thank you.
Big Data
Region: “Big data” has been in the news a lot lately. Some might wonder if it’s a fad, in a sense, given all this publicity. Because you’ve done a wide range of work from deep theory to applied economics using large databases, your perspective on this would be valuable. And, of course, you’re deeply involved in the Internet and its dynamics. What are your thoughts about big data? Does it portend, as some have suggested, an “end to theory”?
Athey: Absolutely not an end to theory. In fact, the need for theory is in some ways magnified by having large amounts of data. When you have a small amount of data, you can just look at the data and build your intuition from it. When you have very large amounts of data, just taking an average can cost thousands of dollars of computer time. So you’d better have an idea of what you’re doing and why before you go out to take those averages. The importance of theory to create conceptual frameworks to know what to look for has never been larger, I think.
Region: And yet some have argued that because data exist in increasingly large quantities, all you really need is to “see what the data say.”
Athey: I think what is true is that when you have large amounts of data, if you ask it the right questions, you have a greater ability to let the data speak, and so you can be much less reliant on assumptions. But you still need a strong conceptual framework to understand what’s coming out.
And I would say in the business world, this is where there’s an enormous scarcity of talent. I see that there are a fair number of statisticians out there, not nearly enough, but a fair number of data scientists out there. There’s a huge demand for them still.
But among data scientists, the ones who can define a question and introduce a new way of looking at the data—those data scientists are rock stars. They’re pursued by every company and they move up the hierarchy very quickly. They’re giving presentations to top executives and are extraordinarily influential. And there are never enough of them.
I think that the data scientists should take a little more economics. That would help; economics puts a lot of emphasis on the conceptual framework. And I also think that economics should be paying a lot more attention to the statistics of big data.
Right now, economics as a profession has very little market share in the business analysis of this big data. It’s mostly statisticians. We’re just not training our undergraduates to be qualified for these jobs. Even our graduate students, even someone with a Ph.D. from a very good economics department really doesn’t have the right skills to analyze the kinds of data sets that big Internet firms are creating.
Region: But there are economists like you and others with this expertise, who have a lot of grad students, and you’re intensely interested in training them in the right direction, of course. But still, that’s not enough? Do neither students nor enough professors see the opportunity?
Athey: Well, not that many people have the access to the data. We’re not teaching courses that reflect this. We’re a little bit behind. Econometrics, at the undergraduate level, is not appreciated as much as an expertise that’s extremely important for future employment, and we certainly don’t see a lot of economics majors going on to take extra steps beyond what’s required.
But then they don’t seem to realize that that kind of training is really crucial for being successful if they want to work at companies like Google or Facebook or Microsoft, Yahoo or eBay, Twitter or LinkedIn. It’s very difficult to be influential in those companies if you are not very savvy with statistics. So the old sort of economics undergrad who gets an M.B.A. but doesn’t know a lot of statistics? A few people with that kind of background will be successful at these large tech firms, but they’re going to be handicapped.
I really think we need to make some changes in education. What happens at the top Ph.D. programs isn’t going to really impact the overall workforce. But what we do at the undergraduate level and whether we start offering more advanced or master’s level courses becomes more important—because, really, with just an undergraduate degree it’s hard to be very successful on the technical side at any of these firms.
The question is, how can economics reach a larger set of people? And, again, why is that important? It’s because the economic intuition helps you ask the right questions of the data, which is extremely important.
I guess one other part of your question was, is big data a fad? It’s not a fad; it’s a fact. Companies in all sorts of different industries are starting to generate large amounts of data. The Internet companies were built from the ground up on that data. Other companies are just starting to think about what they do with the data.
If you think about these kind of general purpose innovations like the computer, it took us a while to figure out what to do with the computer. It replaced the secretary and the typewriter, but it took another 15 years before the personal computer really changed the way we do commerce, which you would say really comes with the Internet and businesses being built around it.
With the big data, of course, the Googles and the Facebooks and so on were born on that. But if you take, say, a car manufacturer that might be getting real-time information from monitoring devices within the cars, there’s a first level of things you can do with that data. Like you can look at aggregate failure rates, or something, for certain types of things. You can identify problems.
But there’s a whole other level of optimizations that can be done. And I think that idea will apply across many industries. They’ll start with just the basics of, let’s figure out how to prioritize problems. For example, with software you can get telemetry data about, where are the bugs? What’s causing crashes? That’s sort of the first level of what you do with data: You use the data to identify problems and make priorities. The more frequent the crash, the higher you prioritize in fixing that problem.
But there’s a next level, which includes real-time machine learning, customization, personalization, optimization, where industry as a whole is just inventing what to do with it. And there could be some really radical breakthroughs in different industries. They’re just very hard to anticipate as they start to use these data.
The Internet and the Newspaper Industry
Region: Let me ask about one industry in particular that you’ve studied recently: the newspaper industry. Your initial title for one such paper was “Will the Internet Destroy the News Media?” You’ve softened that some, I think, in a subsequent draft. But how has the Internet affected newspaper advertising, and therefore industry revenue? And to what degree is the industry responding in a way that might assure its survival?
Athey: Well, it’s fairly clear to everybody that the advent of the Internet has been very bad for newspaper profits and that online advertising revenue has not been nearly enough to replace the loss from advertising in the traditional print. And that, furthermore, just the advertising revenue per newspaper bought has gone down a lot.
My research has focused on just a few aspects of that. One of the things I focused on both theoretically and empirically is the effect of the Internet on loyalty—the fact that the Internet makes it much, much easier to switch across news outlets. I’ve shown empirically that news aggregators cause users to greatly broaden the set of outlets they look at and to become much less loyal to their old favorites.
And then I’ve studied what the implications of that switching are for advertising markets. That switching, it turns out, should theoretically lower equilibrium advertising prices. That suggests that those advertising dollars are gone and they may not come back. You can’t just hope that eventually the dollars will follow the readers. Rather, the new medium has changed the competitive conditions and the real fundamentals of the market in a way that’s going to lead to less of the surplus created from advertising accruing to the newspapers.
Region: Which previously had a fairly captive market, a specific geographic audience.
Athey: Right, the newspapers had a pretty good deal before. They generally had exclusive access to a lot of users. That’s a pretty good position to be in, and that’s unlikely to come back. There are a lot of interesting questions, which I haven’t answered but that the industry is grappling with, such as, what are the best business models to adopt in the face of this? And how can we preserve journalism?
Another question that I think we’re going to confront as a society is, how are the new ways of consuming news affecting the types of news that are being produced? So if your news is getting curated through social media and through news aggregators rather than through the editorial page of a major newspaper, the incentives for the creation of that content are fairly fundamentally altered. The returns to establishing reputation for quality are altered. And so the incentives for producing cute pet videos might go up at the expense of international news coverage, which might not be shared as well on social media, for example. So, I’m in the process of trying to study that empirically, but that’s still a work in progress.
Region: We look forward to seeing the results. Thank you.
Endnote
1 On Feb. 28, 2013, a nurse at a senior living facility in Bakersfield, Calif., refused to give CPR to an elderly woman despite repeated requests from a 911 dispatcher, citing a facility policy prohibiting staff from doing so. The woman died later that day. See the blog.
More About SUSAN ATHEY
Current Positions
Professor of Economics; Professor of Economics (by courtesy), School of
Humanities and Sciences, Graduate School of Business, Stanford University,
since 2013
Visiting Researcher, Microsoft Research, New England, since 2008
Consultant to Microsoft Research as Chief Economist, since 2007
Research Associate, National Bureau of Economic Research, since 2001
Principal, Market Design Inc., since 2001
Previous Positions
Professor of Economics, Harvard University, 2006-2012
Holbrook Working Professor of Economics and Professor (by courtesy), Graduate School of Business, Stanford University, 2004-06; Fellow, Center for Advanced Study in the Behavioral Sciences, 2004-05; Associate Professor of Economics, 2001-04; National Fellow, Hoover Institution, 2000-01
Castle Krob Career Development Associate Professor of Economics, Massachusetts Institute of Technology, 1999-2001; Castle Krob Career Development Assistant Professor of Economics, 1997-99; Assistant Professor of Economics, 1995-97
Visiting Assistant Professor of Economics, Cowles Foundation for Economic Research, Yale University, 1997-98
Professional Activities
Member, Nominating Committee, American Academy of Arts and Sciences,
since 2011
Member, President's Committee for the National Medal of Science (presidential appointment), 2011-13
Co-director, NBER Working Group on Market Design, since 2009
Co-organizer, NBER Information Technology/Productivity Summer Workshop, since 2009
Council Member, Game Theory Society, elected 2009
Associate Editor, B.E. Journals in Theoretical Economics, since 2000
Advisory Committee on Editorial Appointments Member, American Economics Association, 2011
Executive Committee Member, American Economic Association, 2008-10
Council Member, Econometric Society, 2007-10
Associate Editor, Theoretical Economics, 2005-11
Economics Panel Member, National Science Foundation, 2004-06
Honors and Awards
Society for the Advancement of Economic Theory Fellow, 2013
National Academy of Sciences Fellow, elected 2012
Honorary Degree, Duke University, 2009
American Academy of Arts and Sciences Fellow, elected 2008
World Economic Forum Young Global Leader, selected 2008
John Bates Clark Medal, 2007
Econometric Society Fellow, elected 2004
Richard E. Guggehime Faculty Scholar, Stanford University, 2004-06
Elaine Bennett Research Award, 2001
Publications
Published numerous articles in top economics journals on a broad range of topics, including industrial organization, econometrics, microeconomic
theory and game theory. Recent publications focus on Internet economics,
auction theory and statistical analysis, and market design.
Education
Stanford University, Ph.D., 1995
Duke University, B.A. (magna cum laude), 1991