In December 2014, Robert Litterman and his wife, Mary, were nearly killed in a car accident. A flaming fuel tanker was hurtling toward them on a New Jersey highway. Mary saw it first and shouted. Bob slammed on the brakes. “This true story illustrates an essential element of managing risk: Time is of the essence,” he later wrote in an eloquent essay on climate change. “You brake hard and fast; you don’t ease on the brakes.”
Passion is not the adjective most often associated with economists. For Litterman, few words are more apt. That essay conveys his energy, and his wide-ranging accomplishments embody it. He has a profound gift for devising advanced quantitative techniques that blend theory and data to solve otherwise impenetrable problems.
He has brought this talent to bear in macroeconomics, finance, and now the environment, and he has made groundbreaking and highly influential contributions to each field. At central banks, investment firms, and increasingly at environmental organizations, Litterman models are fundamental tools.
As an economist at the Minneapolis Fed in the early 1980s, he refined the application of vector autoregression, a method pioneered by his adviser and later Nobel laureate Christopher Sims, to economic forecasting and policy analysis. “Bob is just brilliant at taking ideas—even pieces of ideas—from a conversation and turning them into practical working code,” Sims recently recalled. “Brilliant. And fast.” Litterman’s fluency in code—language that few economists spoke at the time—shaped concepts into quantities, bringing practical life to abstract theory.
Later, as a “quant” at Goldman Sachs, he co-developed the innovative Black-Litterman global asset allocation model that has, since the early 1990s, guided asset managers and financial advisers in crafting investment portfolios. He later created advanced risk management techniques that investment banks rely on still.
And today, while working at Kepos Capital, a New York investment firm, he is deeply involved in climate change economics, hoping to safeguard our most valuable asset. His approach breaks with conventional models by highlighting the centrality of uncertainty and arriving at a call for far higher carbon prices, as soon as possible.
His accomplishments have been widely lauded. He was named the 2013 Risk Manager of the Year by the Global Association of Risk Professionals and in 2012 became the first recipient of the Sussman fellowship at MIT’s Sloan School. In 2008, he received the prestigious Molodovsky Award for outstanding contributions to investment research, an honor given to five Nobel laureates immediately before and after him.
His recent involvement in climate change economics is recognized by his standing on numerous boards, including Commonfund, Resources for the Future, the Robert Wood Johnson Foundation, the Sloan Foundation, and the World Wildlife Fund.
Still, as a risk manager, he is cautious and modest by nature. “I was very lucky in my career to have worked with some very smart people,” he said in a 2013 lecture. But his humility belies the significance of his skill at creating substance from intuition. He’s optimistic that he can help do the same for carbon pricing and that strong remedies become reality before worst-case scenarios do.
Interview conducted July 31, 2019.
Why economics?
Region: I’m very curious about why you became an economist. You majored in human biology at Stanford and then entered a very rigorous economics program at the University of Minnesota. Biology to economics is an unusual academic leap. What intrigued you about economics, and econometrics in particular?
Litterman: Well, in fact, before human biology, I was a physics major. I was interested in science early on and thought I’d eventually be a scientist. The advanced physics program at Stanford, though, was very narrow: very heavy math and physics, just one elective over four years, only 12 students. And it was the height of the Vietnam War, and I just wasn’t entirely sure I wanted to be a physicist in that place and time. So I switched to the human biology program. It was a brand-new program then, and it’s still there. It’s the most successful interdisciplinary program they’ve ever had.
The program was about studying humans as a social animal, a very broad program—biology, psychology, anthropology, history, economics. One of the things that I picked up is that if you want to understand human behavior, you’d have to understand incentives. And that’s economics.
Now, at the time, I was working for the Stanford Daily, the student newspaper, and was very interested in journalism and communications. At that point, I thought I was going to be a journalist. While at Stanford, I was a stringer for Time magazine and then I became an intern at the San Jose Mercury. And my first job was as a reporter for the San Diego Union.
But I started thinking about school again, and particularly about computing. I got into the economics program at the University of California, San Diego. It was a mathematical program, which I hadn’t realized when I applied, but I’ve always been good at math, so it didn’t intimidate me. I met my wife there, and after a year at San Diego, she decided to go back home to Minnesota. I followed her back and applied to the University of Minnesota, not knowing anything about the department. I just thought, “My girlfriend is here; let me see if I can get in.” At that point I was kind of hooked on economics.
Region: When was this?
Litterman: I graduated Stanford in ’73, so this would have been ’75, because I spent a year as a reporter and then a year in San Diego. So ’75.
Region: This was definitely not a straight academic track.
Litterman: No, not at all. I wasn’t planning to be an academic. But when it was my turn to go into the job market, one of my advisers, Tom Sargent—the other was Chris Sims—asked me where I’d like to teach. I told him I wasn’t interested in teaching and planned to go into business. But he made a good argument. He pointed out that if you want to be an academic, you can’t start in business. But if you go into academia, you can always go into business. So I did go on the market and was very successful.
But during my first year, I worked at the university’s offsite computer center. When I interviewed with Neil Wallace, he said, “We usually have one of our graduate students work at the university computer center. Do you know any programming?”
I did a little FORTRAN, kind of self-taught, so I said, “Sure, I’ll be happy to do that.”
That was very beneficial. I learned a lot of programming. I supported two large statistical packages, BMDP and SPSS, and learning how to support a large computer program was very useful. It was also really nice to basically have an unlimited computer budget.
Vector autoregression
Region: I know that you spent a lot of that computer time studying vector autoregressions, so let’s talk about that work. Your dissertation on VAR was completed in 1979.
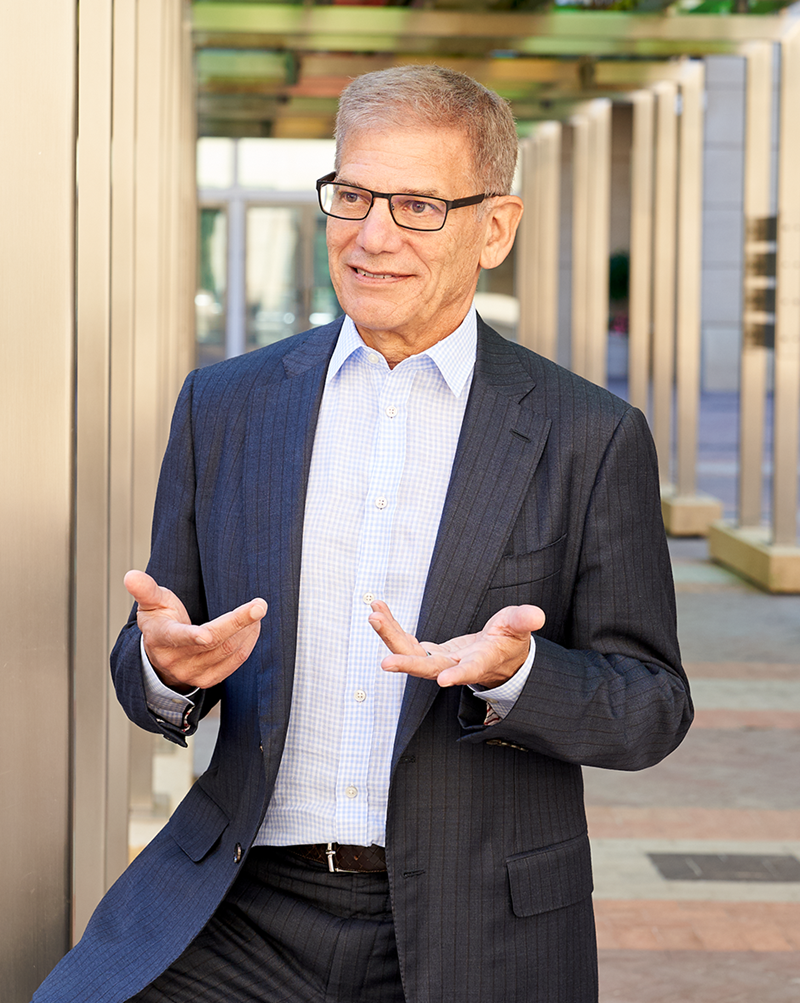
My understanding is that VAR is basically a technique that estimates a current variable by using its past values and those of related variables. And it can then do the same for those related variables. So it can be used to estimate future inflation, for instance, by using current and past values of inflation, and also values of unemployment and GDP growth—and then be used to forecast unemployment and GDP growth.
Could you elaborate on the technique?
Litterman: In those days, a lot of economic forecasting, including the Minneapolis Fed’s, was done with large models that had, in many cases, hundreds of equations. Economists were not happy with them; they often generated forecasts that made no sense. Users typically ended up saying, “Well, we have a pretty good sense of what we want the GDP forecast, say, to look like, so we just have to come up with GDP components that add up to that.” And then to get a reasonable forecast, the economist would put in what are called “error terms” or sometimes “fudge factors.”
So the models themselves really weren’t providing useful forecasts, and you’re thinking, “What value is coming out of this when I have to tell the model what the answer is?” There were important theoretical objections to them as well, based in part on rational expectations and the idea that, as Chris Sims put it, these large structural macro models required “incredible assumptions” about how people will react, in a very narrow way without taking into account available information.
At the same time, folks at other schools were saying, “There’s a very simple way to forecast. You just project a variable on its past history and hope it’ll look similar going forward.” It was extremely simple, really no substance to it at all, and yet it was hard to beat. You might run a regression of a variable on its past value. Or maybe there’s a second lag. Very simple: You only look at its own past and a very short history.
Now, the idea of vector autoregression was that maybe inflation also depends on money supply or output or unemployment. Chris had pioneered this work before I got to the university. He had written a paper called “Macroeconomics and Reality” when I was a graduate student, and folks at the Minneapolis Fed were interested in exploring its ideas, particularly vector autoregression.
Now, in my first year at Minnesota, I was taking Tom Sargent’s macro class, and I used a computer to solve a problem he gave us. I think he loved that skill and said, “You know, Bob, maybe you could come work for me at the Fed as a research assistant.”
I got that job at the Fed, and a year or two later, folks there asked me to help them in this forecasting exercise and suggested maybe trying to use a vector autoregression, as had been suggested by Chris. The first thing I did was program up a vector autoregression, estimate it, make some forecasts looking at standard variables like inflation, money supply, output, unemployment, government spending, and so on.
Region: And it worked better than the large macro models they’d been using?
Litterman: No, it worked terribly! A simple random walk did a better job without estimating anything. I went back to Chris and said, “These vector autoregressions do a terrible job of forecasting.” And he said, “Well, have you tried Bayesian priors?”
Region: And you use a Bayesian approach to limit the problem of too many parameters. That’s the problem you needed to solve.
Litterman: Right. Chris’ suggestion was to use a Bayesian prior to limit the overparameterization. The bad forecasts were basically from trying to estimate too many parameters without enough data. We were fitting noise in the historical data and then projecting forward on the basis of noise.
Region: You’re looking for a very weak signal amid a lot of noise.
Litterman: Exactly.
Region: And the Minnesota prior, or I’ve heard people call it the Litterman prior—
Litterman: True, people call it the Litterman prior, but let’s be very clear, it was Chris’ suggestion. He proposed using a Bayesian prior. I said, “What should I use?” He suggested maybe just a random walk. So that was definitely Chris’ idea. All I said was, “All right, sounds good to me. I’ll try it.”
Region: That’s very modest of you.
Litterman: I was shocked at how well that worked. Well, let me be careful when I say how “well” that worked.
When you just use a standard regression, without a prior, errors in the forecasts are very big. Very bad performance. With a prior, you could shrink toward this random walk idea and get improvement. So the right way to think about it, really, is to start from that random walk prior and then let the data pull you a little bit away. With that approach, you can do better. But if you go too far, things get worse. It was better than the simple univariate regression; you could pick up some interesting dynamics both from the own values previously and then also from the other variables. So they were helpful at the margin, but you couldn’t let the data speak too loudly or the noise would overwhelm the signal.
It didn’t take me long to program that up, test it out, and see how it worked. Chris said, “Write it up, and you’ll be done.” That was my thesis. I was very lucky as a graduate student because I had been pointed at a very promising approach.
Region: You graduated in 1979 and went to MIT for a couple of years before returning to the Fed, so you did your academic stint, as Tom suggested.
Litterman: Yes, I did my academic stint. And, again, I was very lucky in the job market. My thesis was really just one topic: “Techniques of Forecasting with Vector Autoregressions,” and it was very well-received on the job market. It was relatively easy to understand and very practical. But I also have always said that I was very lucky because I came out of the University of Minnesota a year after Lars Hansen, and Lars was, in the eyes of many, the best economist of our generation. He came out of Minnesota a year ahead of me, so a year later, the job market was looking for Minnesota grads.
I got a lot of good offers and ended up going to MIT. It was probably the lowest-paying school. You know, in those days, the more prestigious the school, the less they paid you. They told us we were getting paid in human capital! Well, after two years, I was tired of being paid in human capital.
And, to be honest, the intellectual atmosphere at Minnesota, both at the Bank and at the university, was great. I could now compare MIT and Minnesota, and I was happy to go back to Minnesota, especially to the Bank. They had so many interesting problems, and I could spend my time doing research; whereas, at MIT I was spending a lot of time teaching, and I wasn’t particularly good at it or interested in it. I was still interested in figuring out how the world works.
Acceptance of VAR forecasting
Region: MIT’s loss was clearly Minnesota’s gain. At the Minneapolis Fed, your VAR forecasts became an essential part of [Minneapolis Fed President E.] Gerald Corrigan’s preparation for Federal Open Market Committee meetings.
Litterman: Yes.
Region: And you said your job market paper on VAR was well-received in academia. Were other Fed districts and the Board in Washington receptive to VAR then?
Litterman: Not so much, early on. No, it was a bit of an attack.
Region: So there was pushback on it, as there was on rational expectations?
Litterman: Well, we were sort of attacking the basic tool that the Fed relied on and used. They were open to VAR as a new idea out there, you know, in the region. That has always been a good thing for the Fed, I think. One of the benefits of the regional Banks is to allow new ideas to spring up. So I don’t think the Fed pushed back on it, but it wasn’t as if the Board immediately said, “OK, we’ll use this new approach.”
VAR 40 years later
Region: In the 1980 paper you mentioned, “Macroeconomics and Reality,” Chris said that VARs would be useful in a number of roles: in interpreting and identifying data, in forecasting, and also for evaluating policy.
You wrote several papers at the time demonstrating its use in those roles. In 1984, you wrote “Forecasting and Policy Analysis with Bayesian Vector Autoregression Methods” in the Quarterly Review. In 1986, you wrote a couple of papers that reviewed five years of experience using VAR for forecasting, and you said it did remarkably well relative to commercially available forecasting models that cost thousands of dollars. As you pointed out, VAR forecasts could be done on a laptop in 10 minutes, at very little cost.
With 40 years’ perspective, how do you think VAR has stood up in these roles? Not just in forecasting but especially in evaluating policy, which seems to be viewed more skeptically.
Litterman: Yes, policy analysis is difficult with VAR because, at best, VARs tell you about, let’s say, dynamic correlations.
But there’s a number of problems. One problem is that the economy is not really fixed. It’s an evolving system. The dynamics that might’ve been true 40 years ago might be very different from the dynamics today. You’re always using history to forecast the future. You only have the history.
Region: Can’t you use time-varying coefficients to account for that change?
Litterman: Yes, you could. Actually, one of the chapters in my dissertation was: Let’s see what happens if we use time-varying coefficients. Well, it turns out that if you don’t put any structure on the problem, that just multiplies the number of free parameters.
Region: Well, yes, of course. Geometrically.
Litterman: Right. I tried that, and I found that as soon as you start allowing time variation of the coefficients, things get worse. There wasn’t room for even a slight improvement there. That tells you something about the weakness of the signal.
So one issue is that the economy changes over time. It’s hard enough to capture an aggregate useful signal, much less how it’s going to change when you change policy. You really need structure to do that.
The paper I wrote about trying to use VARs for policy analysis basically said that if we think of policy as a random shock and that the Fed is every-so-often randomly shocking the economic system, then we can look at how that shock impacts the system, assuming everything else is the same.
Well, the assumption that everything else is the same only follows if you think everyone knows that the Fed occasionally randomly shocks the system, so they’re not surprised by this random shock, and it doesn’t provide any new information.
To the extent that people understand what the Fed is trying to do and they’re making sense out of it, and the Fed does something that surprises them, no doubt their expectations are going to change. That’s sometimes called the Lucas critique, right?
And the other problem of treating shocks as policy is, is it just the money supply? Is that what the Fed shocks? Or is it interest rates? Or is it both?
Region: What’s the innovation?
Litterman: Right, what’s the innovation? In a multivariate context, what is a Fed shock?
So there are a number of issues that have always been there and understood with respect to forecasting and trying to do policy analysis with what’s sometimes called an approach without structure or “reduced form,” as it’s sometimes called in econometrics.
The problem I looked at was, suppose what the Fed does is shocks the money supply, or maybe you define it to be an innovation both to interest rates and to money supply. What would optimal policy look like if the objective is, let’s say, stabilizing output and inflation, or something along those lines?
You can bring a rich literature to bear about dynamical systems and having objectives. In a linear quadratic system like that, you can come up with an optimal policy.
Many economists—and I would sort of agree with this—would say that if the Fed were actually to use that approach, then it would no longer be credible that it’s just shocking randomly. It’s actually now systematic, and so the whole thing unravels.
These things are always interesting to look at in terms of the dynamics. When there’s a shock to a certain variable, how does the system respond? That may be indicative of something deeper that we can better understand, so it might inform or suggest questions for research. But actually doing policy analysis? We’ve always understood that that is very difficult to do with these reduced-form systems.
Why move to Goldman Sachs?
Region: You were working at the Fed for five years, and you then went to Goldman Sachs for 23 years, until 2010. Like your move from biology to economics, that seems a large leap, from a district Reserve Bank to a major investment bank.
I imagine part of the appeal was being able to work with Fischer Black, co-author of the famous Black-Scholes options pricing model in 1973. As you later wrote, most economists would probably consider it the most important achievement ever in finance.
Litterman: First of all, I should say that Goldman Sachs came after me, and it wasn’t because of Fischer Black. Fischer did interview me, but I think that if it’d been up to Fischer, I wouldn’t have been hired. [Laughter.] In the interview, he said to me, “So, Bob, you’re an econometrician. What makes you think an econometrician has any value to add on Wall Street?”
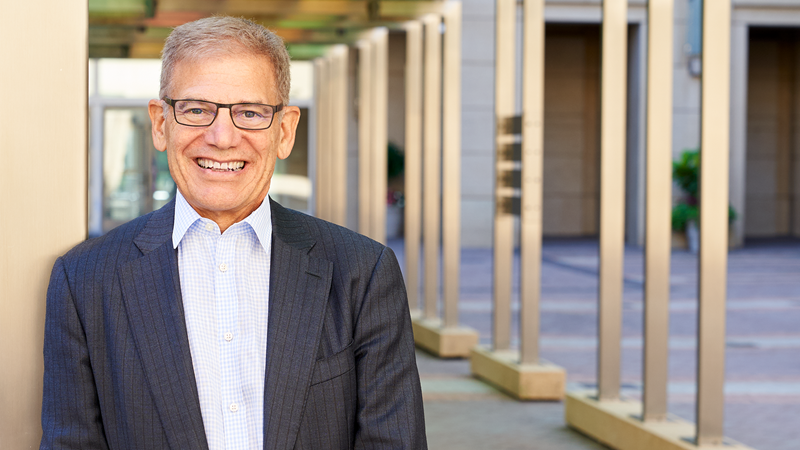
That was a question I was totally unprepared for. After all, I didn’t go to Wall Street; they sought me. And, to be honest, I was very unfamiliar with Wall Street investment banking or what they did or how they made money or how I could add value. They came after me.
Region: Presumably someone at Goldman saw the value an econometrician might add! You were a quantitative analyst—
Litterman: Yes, I was a quant and Fischer was a quant. There weren’t many on Wall Street in those days, so it made sense that he interviewed me. But I don’t think he was convinced I was going to add value, and I certainly didn’t change his mind during the interview. But I got hired anyway.
Black-Litterman model
Region: Together, you two created the groundbreaking Black-Litterman global asset allocation model. My understanding is that model illustrated weaknesses of Harry Markowitz’s mean-variance theory and improved upon it. The theory itself was brilliant, of course. He won a Nobel Prize for it.
But it was virtually impossible to implement. It required asset return estimates for every asset class, and slight changes in those estimates resulted in wholesale changes in allocations that often made little sense—massive shorting of one nation’s currency and going long on another’s, for instance.
So years after he came up with the theory, it still wasn’t being used by investment banks or financial advisers. Your model addressed those weaknesses, and it’s now a standard tool for asset managers.
Could you explain how it does so?
Litterman: I should start by saying that I found Goldman Sachs, and Wall Street in general, to be very rich with interesting problems to work on for clients in those days. Asset allocation was one of those. Interestingly, the model itself was motivated by a desire on the part of our fixed-income colleagues in Japan to help Japanese investors build global fixed-income portfolios. I was in Goldman’s fixed-income area. Fischer was in the equities area. But, as I said, there weren’t that many quants, so I went to talk to Fischer and said I had never used one of these optimal asset allocation models.
Fischer said, “Well, you know, my attitude on these problems is to start out simple, and if it doesn’t work, then you can always do something more complicated. So why don’t you start with the standard Markowitz model?” I programmed up a Markowitz optimization with eight currencies and nine bond markets. A total of 17 variables, but it’s 17-by-17. That’s not huge, but these are highly correlated variables. Then I put in our economists’ forecasts for the exchange rates and the interest rates at a six-month horizon.
I ran the optimization, and what came out looked crazy. It was long 80 percent this and short 120 percent that and, you know, the biggest position was New Zealand or something.
Region: And if you changed the forecasts—
Litterman: Exactly. I thought, “This can’t be right, so let me change these forecasts a little bit, five basis points, something very small relative to the forecast uncertainty.” Well, all of a sudden, you got a completely different portfolio.
I was discovering what had been well-known. But before I went back to Fisher, I spent a full day trying to debug the program. I thought I must have converted five basis points into 5 percent somewhere in the code, something like that. But after I convinced myself that there was no bug, I went back to Fischer and said the standard asset allocation models are incredibly sensitive to the inputs.
And Fischer said, “Yes, that’s well-known. The standard approach is to put constraints in, maximums and minimums.” Well, that reminded me of what the Fed used to do with VARs.
Region: Yes, very similar, a Bayesian approach. Introducing a prior to shrink—
Litterman: Right, very similar. And he said, “I’ve just written this paper called ‘Universal Hedging,’ and it has a global equilibrium in it; maybe we should put that into the model.”
Region: In a sense, that was the prior. And the equilibrium he proposed is sort of a CAPM [capital asset pricing model] equilibrium?
Litterman: Yes, that was the prior. And it was a global CAPM equilibrium, a global version of the standard equilibrium model for assets that says that, in equilibrium, every asset should get paid a return proportional to its contribution to portfolio risk, and that is then equal to its beta [an asset’s covariance with the entire market’s return] with the market portfolio. To be honest, I had previously seen Fischer’s paper and thought it had nothing to do with what I’m working on now.
But when he said, “Let’s put it in our model,” I thought, “OK, let me read that paper, see what it is.” There were a number of differences. Obviously, that’s typically an equity model. I was in fixed income. It’s an equilibrium model. I was working on a problem when you have forecasts. The first thing I had to do is think, “What does it even mean in this context?”
Well, in equilibrium, each asset will have an expected return proportional to its beta with the market portfolio. In this context of portfolio optimization, I quickly realized that, in effect, you can think of that equilibrium return on assets as well as currencies as being a forecast. So now I’ve got an equilibrium return. And I’ve got my economists’ views, and I thought, “Well, yes, that’s a lot like the vector autoregression with Bayesian priors.” I can think of equilibrium as being a prior, and I can shrink from economists’ views toward equilibrium and see if I get a similar improvement in the behavior of the optimal portfolio at least.
I found something very similar but, in this context, a little disconcerting. If you started with the equilibrium, you got a very natural portfolio with market capitalization weights in all the assets and currency hedged as Fischer had suggested. But as soon as you move a little bit away from that and put any weight on the economists’ views, it seemed to go in crazy directions.
What I realized is that you have to take into account the correlations of the assets, because these were highly correlated assets. That was basically the problem. If you just say, “I’m going to change the forecast on the 10-year bond,” and you don’t change the forecast on the five-year bond, a highly correlated asset, the model’s going to say, “Here’s an opportunity to go long on the 10-year and short on the five.”
Region: So that’s why you got big swings in asset holding recommendations when expected returns were slightly changed.
Litterman: Yes, that’s the intuition behind all those big swings. And when you do take the correlations into account, it’s almost like magic. All of a sudden, when the 10-year bonds move up, the five-years should move up this much, the two-years should do this, and so on.
The nice thing about having equilibrium as well as a forecast is you don’t have to have a forecast on everything. I can now say, “I’m bullish on just the 10-year bond. Tell me what that implies.” The model then fills in all the other forecasts in the most consistent way possible. The optimal portfolio is just to move a little bit away from market cap weights to overweight that asset you just said you liked.
Region: So essentially Black-Litterman takes the global equilibrium market as the starting point and then tilts it with investor beliefs, and confidence about those beliefs.
Litterman: Right. Two of the nice things about Black-Litterman are, one, you don’t have to have a view on everything, and most people don’t have views on everything.
Region: And for Markowitz, you did.
Litterman: For Markowitz, you did. You had to put in all those forecasts. With Black-Litterman, you can put in your views. And views can be very general statements like, “I think cheap assets are going to outperform expensive assets.” As a quant on Wall Street, that’s a very interesting way of translating any metric that you think has forecasting ability into a portfolio tilt. Also, you can put different degrees of confidence on those different views.
So the real power of Black-Litterman is just that the inputs become much more intuitive. The optimal portfolios are much more intuitive as well. They tilt in the direction of your views.
Now, if all you’re doing is specifying views and then tilting in that direction, where’s the value added? I mean, you can always say, “I want to overweight the 10-year bond.” So, what’s the difference between saying, “I’m bullish on the 10-year bond,” do an optimization, and it tilts that way, or just saying, “Let’s tilt that way.”
The answer is that the world is never that simple. You always have constraints and transaction costs and an existing portfolio that has certain tilts.
What’s really nice about Black-Litterman is that since you can trust it to do the right thing in a simple context, you can have more confidence that in the real world, which is much more complicated, it’s going to lead you in the right direction.
Region: And its value has been shown by its widespread adoption. It was developed almost 30 years ago, and it’s still the workhorse of global asset allocation. It’s amazing.
Litterman: It is. I really have been amazed by it. I certainly didn’t expect it. I’ve worked on many models that never see the light of day! [Laughter.]
Economics of climate change
Region: Let’s move to climate change economics. What motivated your deep involvement in this issue?
Litterman: What led to my interest was my background in risk management. After I came to Goldman and spent a number of years in fixed-income research working on problems like the asset allocation model, I was asked to move into risk management. Actually, in 1994, Goldman offered me the position of being a partner and head of risk management, which I was delighted to do.
It provided me with quite an education in risk management. We were a partnership at the time, and a partnership is a great framework for managing risk. All of you are in it together, using each other’s money. It’s not other people’s money. It’s our money. There’s a real sense of responsibility.
The first thing I did was ask all the other partners who were managing risk how they did it. It was quite an education. I like to emphasize that I didn’t teach Goldman Sachs how to manage risk. Goldman Sachs taught me.
Later on, one of my partners, Larry Linden, who was head of operations, asked me whether I was interested in the environment and environmental organizations, and what I was planning to do when I retired. This was about 15 years ago, and I was still an active partner far from retirement. But he planted the seed and introduced me to a few folks in the environmental community, including the head of the World Wildlife Fund.
I got interested because like many people, I thought of climate change as a serious problem. But I remember saying to Larry that it was obvious we’re not pricing climate risk appropriately. Larry agreed and said the problem is that no one knows where it should be priced. I remember thinking, “I’m an economist. I know something about risk management. I’m going to go figure this out.”
I soon learned that Bill Nordhaus was the leading researcher in the field. I knew Bill from the days when we were both young macroeconomists, and he had turned to this problem much earlier in his career. But his approach wasn’t consistent with the way we price risk on Wall Street. To be fair to Bill, he started decades earlier when risk pricing really wasn’t understood that well. There was a revolution in how we think about asset pricing, which frankly started with Lars Hansen and others from Minnesota. And Bob Lucas. I read the academic literature on climate change and thought, “This is kind of an asset pricing problem. These damages in the future are uncertain, so we can’t just pick a discount rate.”
One of the problems with Bill’s approach was that he used a constant discount rate. He didn’t look at the full distribution of potential outcomes, which is to say, risk. His approach was to have some sense of damages as a function of temperature. They’re all in the distant future. Let’s discount them to the present. And that expected discounted damage is what we should charge people for putting more pollution into the atmosphere.
Region: It’s very deterministic—no uncertainty about damages or costs.
Litterman: Yes, it’s a deterministic approach. And the problem is that there are many potential outcomes. It could be very bad, or it could be not bad at all. The discount rate that you use depends on marginal utility in those states of nature. That’s when we talk about risk pricing.
That’s how we price risk on Wall Street. And that’s how you need to price climate risk.
One thing I read at the time was an early paper by Larry Summers, whom I knew from MIT, and Dick Zeckhauser at Harvard. They talked about the fact that you can’t assume that more risk increases price, which was my intuition. It turns out not to be so obvious. The price really depends on the covariance of the outcome with marginal utility. To keep it very simple, the idea is that if damages occur in bad states of nature, you want to put more weight on them, while if they occur when things are good, you don’t worry about it so much.
Region: In that sense, it’s like insurance—valuable only when things go wrong.
Litterman: It’s like insurance. Exactly.
Well, Bill Nordhaus, in one of his early papers, had said real growth and pollution are correlated. Stronger growth leads to more pollution, and less growth leads to less pollution. We saw that in the Great Recession: Pollution went down as the economy weakened. There’s nothing wrong with that assumption, but if you assume that when times are good, there will be more pollution and more damages, then the pollution acts as a hedge with respect to economic growth. Therefore, pollution reduces the risk with respect to growth and means that you’re going to lower the price on pollution. Well, to most people, that doesn’t make a lot of sense. They’d say only an economist would come up with that.
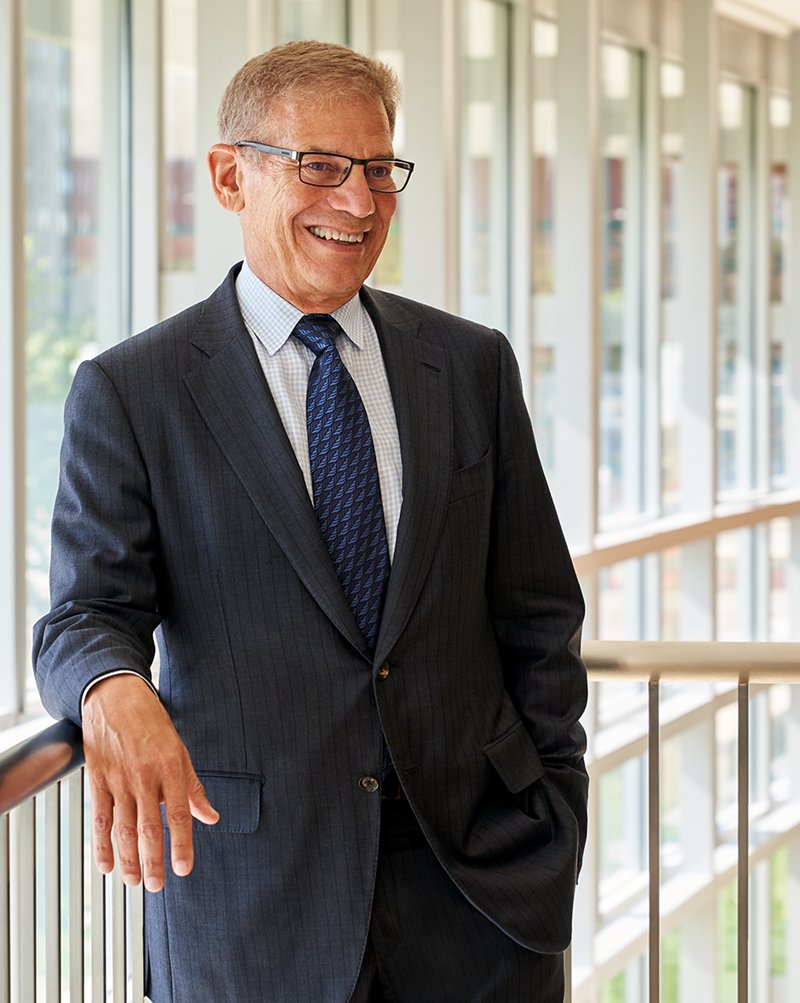
But another way of putting it is, if real growth uncertainty is the dominant uncertainty—and economists tend to think that way—then you get the Nordhaus-type of result. But if you think that there might be really catastrophic risk from climate change, then that could be the dominant source of risk. So, if the bad outcome is a bad climate outcome, then of course you want to have a higher price.
Region: That’s pricing for extreme consequences.
Litterman: It’s really pricing for the worst-case scenarios. One of the sort of obvious lessons of risk management is you have to worry about worst-case scenarios. We’re not pricing climate risk because of the expected outcome. We’re pricing it because it could be much worse than what we anticipate. There is tremendous uncertainty about the future.
Sadly, from a political perspective, that uncertainty has been used as an excuse not to do things. “Let’s wait and learn more” is the argument. Well, if the uncertainty could be really bad, then that uncertainty is a source of risk, and you want to act sooner.
Region: My understanding is that Nordhaus’ DICE model starts at a fairly low price, maybe $5 per ton of carbon, with a possible rise in the future. Your EZ-Climate model with Kent Daniel and Gernot Wagner reaches the opposite conclusion: a high initial price with a potential decline as technology advances and the situation is clearer. Could you elaborate on that difference?
Litterman: Yes, Nordhaus’ model starts with a low price, and it increases over time. That’s sometimes called a policy ramp. I like to call it “easing on the brakes” because if we think about it as driving a car and there’s a cliff up ahead, we know we’d better slow down at some point. Well, if you know where the cliff is and how much time you have, then you can ease on the brakes.
But if you don’t know how far it is, if you’re driving in a fog, you don’t ease on the brakes. You slam on the brakes. That’s the intuition. And the price we charge for emissions is the brake. As an economist, I would say it’s certainly the main brake we have. So, if we have more uncertainty, we want to have a high enough price that we’re very confident we’re going avoid that worst-case scenario.
If we estimate a carbon price of about $120 per ton, that translates to $1 plus per gallon of gasoline. But there are many cheap ways to produce energy—solar and wind, for example. At $120 per ton of emissions, there is going to be a rapid decarbonization of the economy. People will continue to drive their cars, but the transition to electric vehicles will be that much faster.
The electricity sector will be decarbonized very quickly. People will insulate their homes. Energy demand will be lower than otherwise. As an economist, I see this a little bit like gravity. If gravity is pulling you down, it’s going to be hard to move against it. Incentives are very fundamental. We’ve got to change behavior, so we’ve got to change incentives. And when we do, that’s going to change the behavior of every individual in the world without their even knowing why.
Is a high carbon price realistic?
Region: Is it realistic to assume that nations, including the United States, will be willing to charge such a high price? My sense is that people don’t seem ready to slam on the brakes.
Litterman: Well, there’s the political issue versus the pure economics of the problem. Our model addresses the economics. The politics wouldn’t be as difficult if the money is returned to individuals. I sit on the board of the Climate Leadership Council. We propose a carbon dividend plan that gives back the tax revenues in a lump-sum payment. It’s a very progressive plan: About 70 percent of people would be better off. People who don’t use much carbon are going to benefit as opposed to people like me. I have a large carbon footprint because I fly frequently, and airplanes use a lot of carbon. So I don’t think it’s that difficult politically.
What makes it difficult politically is that there are winners and losers from pricing carbon. The winners are mostly not represented at the table. They are the future generations: my grandchildren and their grandchildren and their grandchildren’s grandchildren, none of whom are here voting today. They’re the ones who are going to be made better off by this policy.
A lot of people are very pessimistic about action on climate right now because of the political situation in Washington. But I’m actually much more optimistic because of changes by the oil industry, the biggest industry at risk from appropriate addressing of climate change.
Just two months ago in June, the heads of all the major global oil companies—Shell, Exxon, BP, Total, et cetera—20-some CEOs met with the pope and signed a statement supporting pricing of carbon. And not just the CEOs but their shareholders were represented. So you have management and owners promising to work toward this.
You still have some pockets of resistance—the American Petroleum Institute, for instance. And I haven’t heard the Koch brothers come out in favor of pricing carbon yet. There are a few others who haven’t yet got the memo.
But look at the Climate Leadership Council. Our founding members include ExxonMobil, Shell, and other oil majors, as well as GM, Unilever, J&J. It also includes the Nature Conservancy, the World Wildlife Fund. So we have everyone from ExxonMobil to the World Wildlife Fund. How many people are not in that coalition? That’s pretty much everyone.
We know what we have to do. We have to price emissions; we have to change incentives. We’ve got a coalition of players. So I’m more optimistic than most.
Having said that, we should have done this 20 years ago. The risk from climate change is exploding. We’re right now in a world that is about one degree centigrade warmer than historical temperatures, and we’re already seeing huge impacts. No matter what we do, given the lags in the system, we’re probably going to get to two degrees C even if we were to price emissions at a high level today.
We don’t have 10 years to spare. We don’t have three years. This should have been done long ago. Carbon pricing is the only brake we have, and we’ve got to slam on it immediately.
Causes of the financial crisis
Region: Let’s move to another crisis, the global financial crisis. You have a unique perspective on it. You were working for an investment bank at the time, but with a background as a central bank economist.
Litterman: And I had been head of risk management—although during the financial crisis, I sat on Goldman’s risk committee but was no longer head of risk management.
Region: What is your sense of the cause of the crisis? And do we have sufficient safeguards in place to prevent another?
Litterman: We could have a long conversation about just this! But obviously, in retrospect, I think the heart of it was mortgages and the lack of appropriate appreciation of the systemic risk in mortgages. Wall Street was able to package up mortgage debt into mortgage bonds, which were viewed as very safe AAA investments and in many cases put in off-balance-sheet vehicles that were viewed as very, very safe.
Region: Why were they considered safe? Misjudgment by rating agencies?
Litterman: There were definitely errors made by rating agencies, but if we take a step back, a mortgage bond has to have enough collateral to cover the payments that come out of that mortgage bond. The rating agencies had a role of saying, “To make this AAA and safe, you have to have this much collateral, and it has to be a mix of different locations and a number of other requirements.”
When I came to Wall Street, we had analysts who would try to find the appropriate mortgages to put into a bond. There was a group of academics at Goldman who said computers can do a better job than analysts at finding the cheapest collateral to back a bond. You can imagine what happened. In effect, you said, “Let’s find the worst mortgages that are sufficient to get this rating given the rating agencies’ requirements.”
And people were going from Wall Street to rating agencies and back and forth. It was almost a game being played. No one really thought these mortgages were going to blow up at first. But over time, tremendous demand developed for these mortgage bonds and, therefore, for the mortgage collateral. So the banks that were providing the mortgages started lowering their standards. Different mortgages weren’t as safe as they had been. Underwriting standards got weaker.
Basically, to satisfy new demand for mortgages, worse and worse collateral was the minimum acceptable to the rating agencies. I wouldn’t point only at the rating agencies. In retrospect, you can see how this whole ecosystem developed. And those mortgage bonds were going off balance sheets, so no one was viewing the risk.
Region: Have adequate safeguards been taken to prevent another crisis?
Litterman: Absolutely. The banking system is much better collateralized, and the oversight by the regulators is stronger. We’re not going to have the same financial crisis. The next one will come out from a different area.
And to be clear, one of the things that the regulators are now worrying about is climate change. I don’t think it’s likely that climate change is going to cause a financial meltdown. I think the financial system is much stronger today than it had been, with more safeguards. That doesn’t mean that something bad won’t happen, of course. This isn’t my area of expertise, but one of the things I worry about is that we may not be able to stabilize the economy as we did after the last crisis. People now tend to expect that the Fed is always going to be there.
Region: The lender of last resort.
Litterman: Yes, lender of last resort. But I think that the Fed and others who worry about financial stability are doing a much better job today. That’s the bottom line.
Region: Those are reassuring words. Thank you.